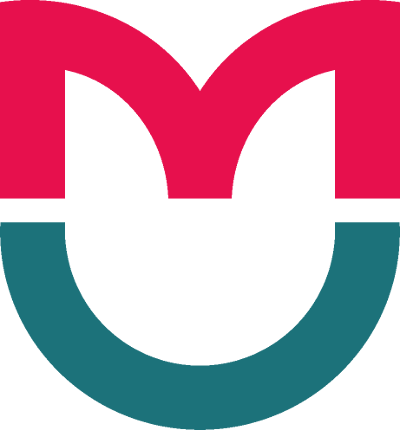
METHOD
Improving eye-brain-computer interface performance by using EEG frequency components
1 Department of Neurocognitive Technologies, Kurchatov Complex of NBICS Technologies,National Research Centre Kurchatov Institute, Moscow, Russia
2 Kurchatov Complex of NBICS Technologies,National Research Centre Kurchatov Institute, Moscow, Russia
3 Faculty of Cybernetics and Information Security,National Research Nuclear University MEPhI, Moscow, Russia
Correspondence should be addressed: Sergey Shishkin
pl. Akademika Kurchatova, d. 1, Moscow, Russia, 123182; ur.liam@nikghsihsgres
Funding: this work was partially supported by the Russian Science Foundation, grant no. 14-28-00234 (acquisition and preprocessing of experimental data), and the Russian Foundation for Basic Research, grant no. 15-29-01344 (evaluation of wavelet features significance for classification).


