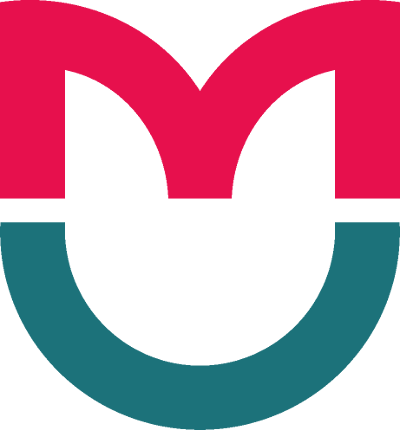
METHOD
Improving eye-brain-computer interface performance by using EEG frequency components
1 Department of Neurocognitive Technologies, Kurchatov Complex of NBICS Technologies,National Research Centre Kurchatov Institute, Moscow, Russia
2 Kurchatov Complex of NBICS Technologies,National Research Centre Kurchatov Institute, Moscow, Russia
3 Faculty of Cybernetics and Information Security,National Research Nuclear University MEPhI, Moscow, Russia
Correspondence should be addressed: Sergey Shishkin
pl. Akademika Kurchatova, d. 1, Moscow, Russia, 123182; ur.liam@nikghsihsgres
Funding: this work was partially supported by the Russian Science Foundation, grant no. 14-28-00234 (acquisition and preprocessing of experimental data), and the Russian Foundation for Basic Research, grant no. 15-29-01344 (evaluation of wavelet features significance for classification).
- Wolpaw JR, Birbaumer N, McFarland DJ, Pfurtscheller G, Vaughan TM. Brain–computer interfaces for communication and control. Clin Neurophysiol. 2002; 113 (6): 767–791.
- BNCI Horizon 2020. The Future of Brain/Neural Computer Interaction: Horizon 2020. Appendix C: End Users. 7th Framework Programme of the European Union. Available from: http://bnci-horizon-2020.eu/roadmap.
- Kaplan AYa, Kochetova AG, Shishkin SL, Basyul IA, Ganin IP, Vasilyev AN, Liburkina SP. Experimental and theoretical foundations and practical implementation of brain-computer interface technology. Bulletin of Siberian Medicine. 2013; 12 (2): 21-9. Russian.
- Kaplan AYa. Neurophysiological foundations and practical realizations of the brain–machine interfaces in the technology in neurological rehabilitation. Human Physiology. 2016; 42 (1): 103–10.
- Chen X, Wang Y, Nakanishi M, Gao X, Jung TP, Gao S. High-speed spelling with a noninvasive brain–computer interface. Proc Natl Acad Sci U S A. 2015; 112 (44): E6058–67.
- Majaranta P. Text entry by eye gaze [dissertation]. Tampere, Finland: University of Tampere; 2009. Available from: http://tampub.uta.fi/handle/10024/66483.
- Jacob RJK. The use of eye movements in human-computer interaction techniques: what you look at is what you get. ACM Transactions on Information Systems. 1991; 9 (2): 152–69.
- Velichkovsky BM, Hansen JP. New technological windows into mind: there is more in eyes and brains for human-computer interaction. In: Proceedings of the SIGCHI conference on Human factors in computing systems; 1996 Apr 13–18; Vancouver, BC, Canada. New York: ACM; 1996. p. 496–503.
- Pfurtscheller G, Allison BZ, Bauernfeind G, Brunner C, Escalante TS, Scherer R, et al. The hybrid BCI. Front Neurosci. 2010; 4: 42. Available from: http://journal.frontiersin.org/article/10.3389/fnpro.2010.00003/full.
- Zander TO, Kothe C. Towards passive brain–computer interfaces: applying brain–computer interface technology to human–machine systems in general. J Neural Eng. 2011; 8 (2): 025005.
- Protzak J, Ihme K, Zander TO. A passive brain-computer interface for supporting gaze-based human-machine interaction. In: Stephanidis C, Antona M, editors. Universal Access in Human-Computer Interaction. Design Methods, Tools, and Interaction Techniques for eInclusion. Springer; 2013. p. 662–71.
- Shishkin SL, Svirin EP, Nuzhdin YO, Fedorova AA, Trofimov AG, Slobodskoy-Plusnin JY, et al. Learn waiting! Contingent negative variation may help you to control with your eye-gaze. In: Pechenkova EV, Falikman MV, editors. Cognitive Science in Moscow: New Studies. Ed. by E. V. Pechenkova, M. V. Falikman. M.: BukiVedi; 2015. p. 486–91. Russian.
- Blankertz B, Lemm S, Treder M, Haufe S, Muller KR. Single-trial analysis and classification of ERP components — a tutorial. NeuroImage. 2011; 56 (2): 814–25.
- Schultze-Kraft M, Birman D, Rusconi M, Allefeld C, Gorgen K, Dahne S, et al. The point of no return in vetoing self-initiated movements. Proc Natl Acad Sci U S A. 2016; 113 (4): 1080–5.
- Ivanitsky GA. Recognition of the task type in the process of its mental solving by a few-second EEG record using the learned classifier. Zh Vyssh Nerv Deiat I P Pavlova. 1997; 47: 743–7. Russian.
- Dat TH, Shue L, Guan C. Electrocorticographic signal classification based on time-frequency decomposition and nonparametric statistical modeling. In: 28th Annual International Conference of the IEEE Engineering in Medicine and Biology Society. 2006. P. 2292-5.
- Roik AO, Ivanitskii GA. A neurophysiological model of the cognitive space. Neuroscience and Behavioral Physiology. 2013; 43 (2): 193–9.
- Frolov A, Husek D, Bobrov P. Comparison of four classification methods for brain-computer interface. Neural Network World. 2011; 21 (2): 101–15.
- Frolov A, Husek D, Bobrov P, Mokienko O, Tintera J. Sources of electrical brain activity most relevant to performance of brain-computer interface based on motor imagery. In: Fazel-Rezai R, editor. Brain-Computer Interface Systems: Recent Progress and Future Prospects. InTech; 2013. p. 175–93.